Predicting clinical antimicrobial resistance
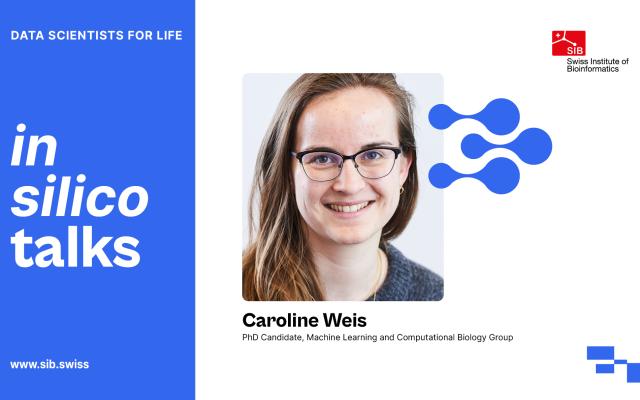
Antimicrobial resistance was named one of the top ten public health threats to humanity by the World Health Organization. To identify microbial species in infected patients, clinicians rely on MALDI-TOF mass-spectrometry and subsequent in vitro experiments. Could machine learning speed up the clinical identification of resistant microbes? Discover the database built by the team for that purpose, and the new method to retrieve even more information from MS data. This should be of particular interest to researchers working in the application of phenotype prediction algorithms, especially on vector represented data, collected across from different domains. The talk is presented by Caroline Weis, former member of the SIB Group of Karsten Borgwardt at ETH Zurich, and the paper discussed in this talk is also one of the SIB Remarkable Outputs of 2021.
About the in silico talks series – The latest in bioinformatics by SIB Scientists
The in silico talks online series aims to inform bioinformaticians, life scientists and clinicians about the latest advances led by SIB Scientists on a wide range of topics in bioinformatics methods, research and resources. Stay abreast of the latest developments, get exclusive insights into recent papers, and discover how these advances might help you in your work or research, by subscribing to the in silico talks mailing list.
Reference(s)
Caroline Weis et al. Direct antimicrobial resistance prediction from clinical MALDI-TOF mass spectra using machine learning. Nature Medicine, 2022.