Whether a gene is expressed or not in an organism comes down to a number of interdependent processes. Among them, the binding of a transcription factor to a short genomic sequence, aptly named “transcription factor binding motif” or TFBM, initiates the transcription. As experimental data are not always available, computational models help researchers predict the location and sequence of these binding sites in genomes. But how well do these models perform? A comprehensive benchmarking study to answer this question has been undertaken by an international team led by researchers at SIB, EPFL and the Russian Academy of Sciences.
Addressing the ‘curse of choice’ of researchers
“Researchers today are faced with a real ‘curse of choice’: there are up to 10 alternative, and often dissimilar, motifs for the same transcription factor”, says Philipp Bucher, Group Leader at SIB and co-lead author on the study. “The need for reliable information on the accuracy of models predicting transcription factor binding sites is thus all the more urgent.”
In an article published in Genome Biology, the scientists addressed the issue of transcription factor binding motif accuracy by benchmarking 4972 motifs from three different resources on 3161 experimental test data sets for human transcription factors generated with three different technologies.
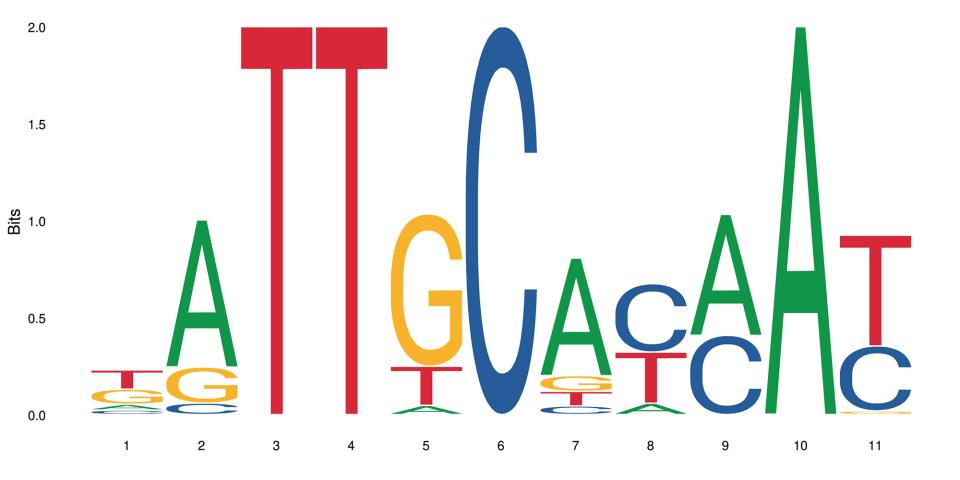
Results and protocols in open access
The complete set of more than 15 million performance values resulting from this all-against-all benchmarking study is freely available from the open access repository Zenodo. To facilitate computational reproducibility, the benchmarking protocols were containerized as docker images and made publicly available from GitHub.
Towards an improved prediction of mutations effects on diseases
The results from this study will help researchers to critically assess published research based on transcription factor binding site predictions. It will also enable them to select optimal motif subsets for particular use cases. “In the long run, we hope that the computational protocols developed for our benchmarking effort will lead to a significant improvement of bioinformatics tools to predict the effects of regulatory genetic mutations in various diseases contexts”, concludes Bucher.
Reference(s)
Ambrosini G et al. Insights gained from a comprehensive all-against-all transcription factor binding motif benchmarking study. Genome Biology 11 May 2020